Abstract
Learned world models summarize an agent’s experience to facilitate learning
complex behaviors. While learning world models from high-dimensional sensory
inputs is becoming feasible through deep learning, there are many potential
ways for deriving behaviors from them. We present Dreamer, a reinforcement
learning agent that solves long-horizon tasks from images purely by latent
imagination. We efficiently learn behaviors by propagating analytic gradients
of learned state values back through trajectories imagined in the compact state
space of a learned world model. On 20 challenging visual control tasks, Dreamer
exceeds existing approaches in data-efficiency, computation time, and final
performance.
Behaviors Learned by Dreamer












Atari and DMLab with Discrete Actions












Multi-Step Video Predictions
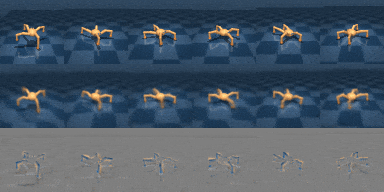
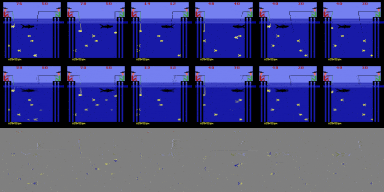
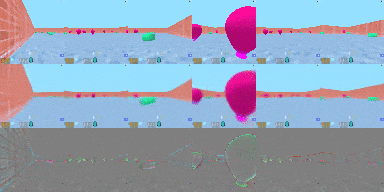
Read the Paper for Details [PDF]
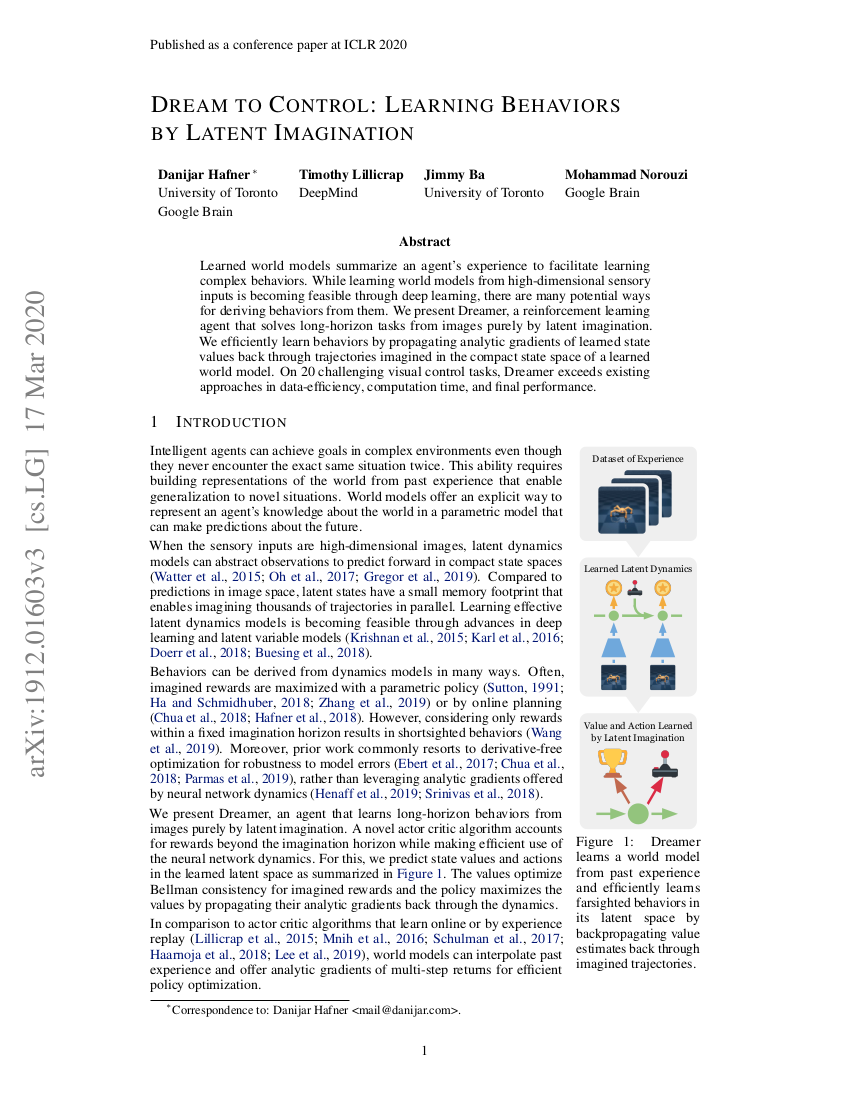
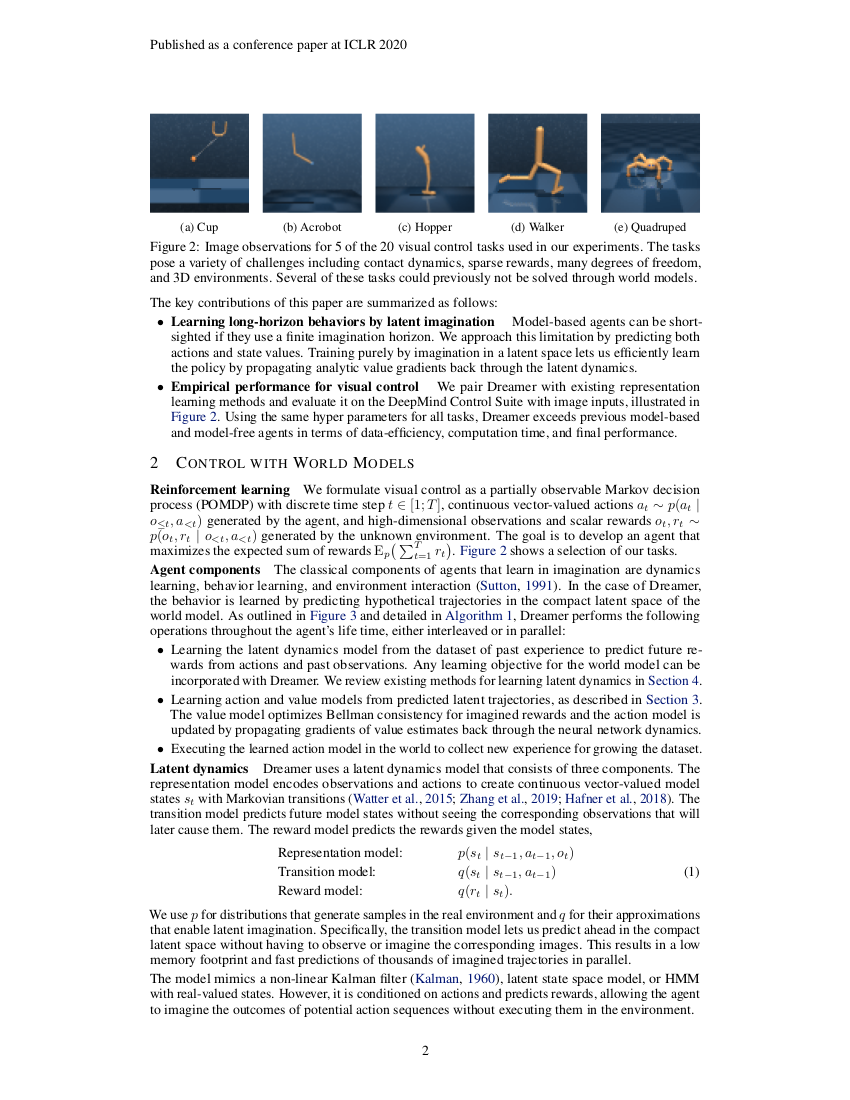
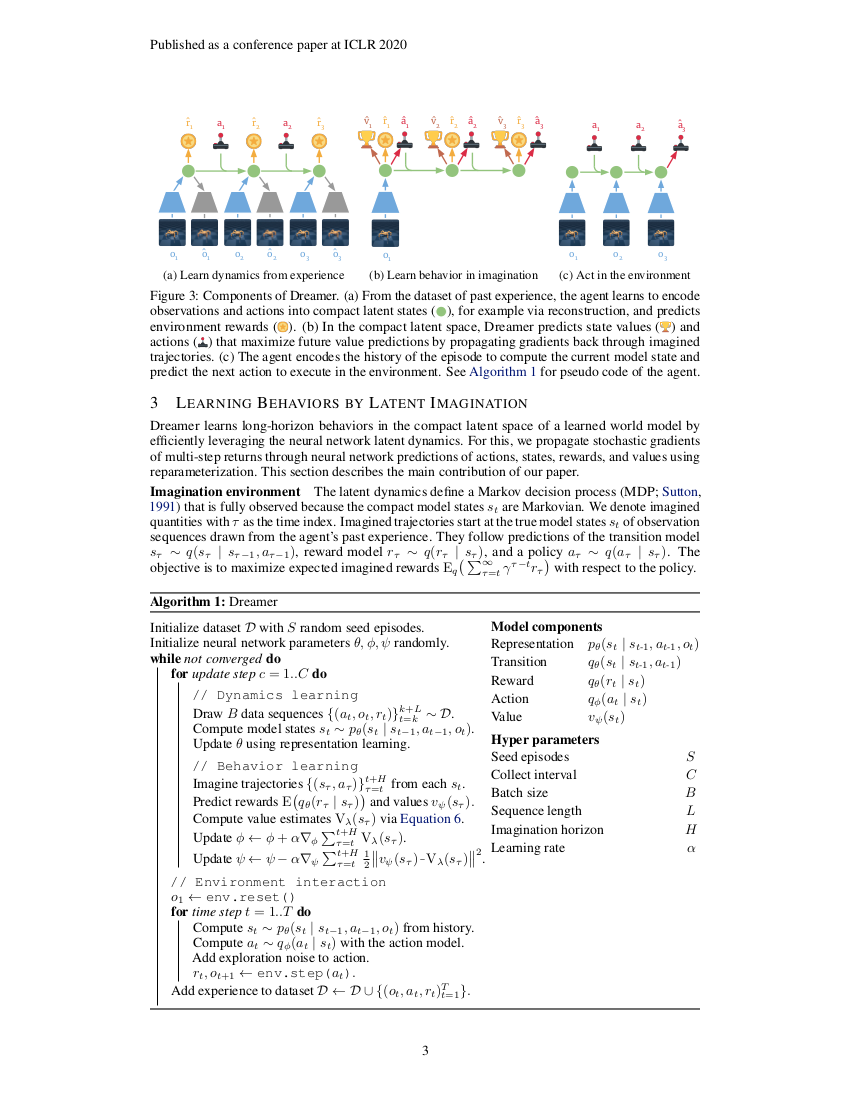
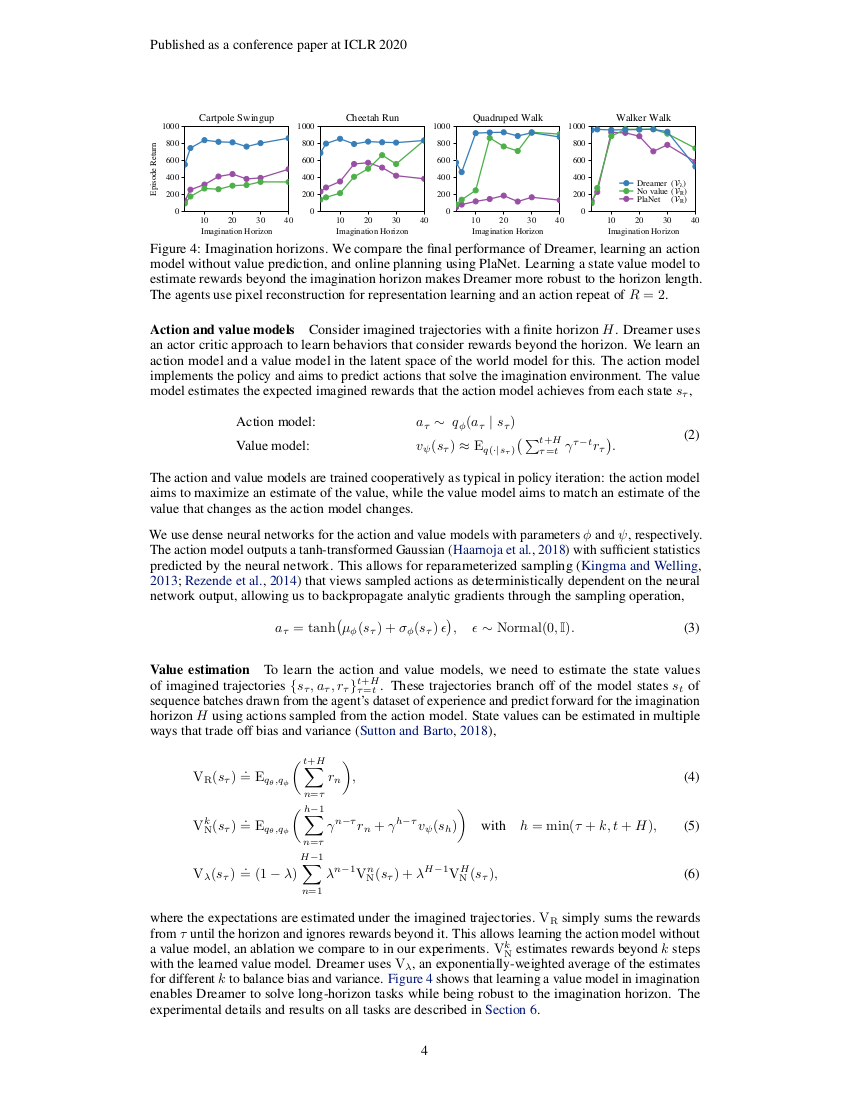
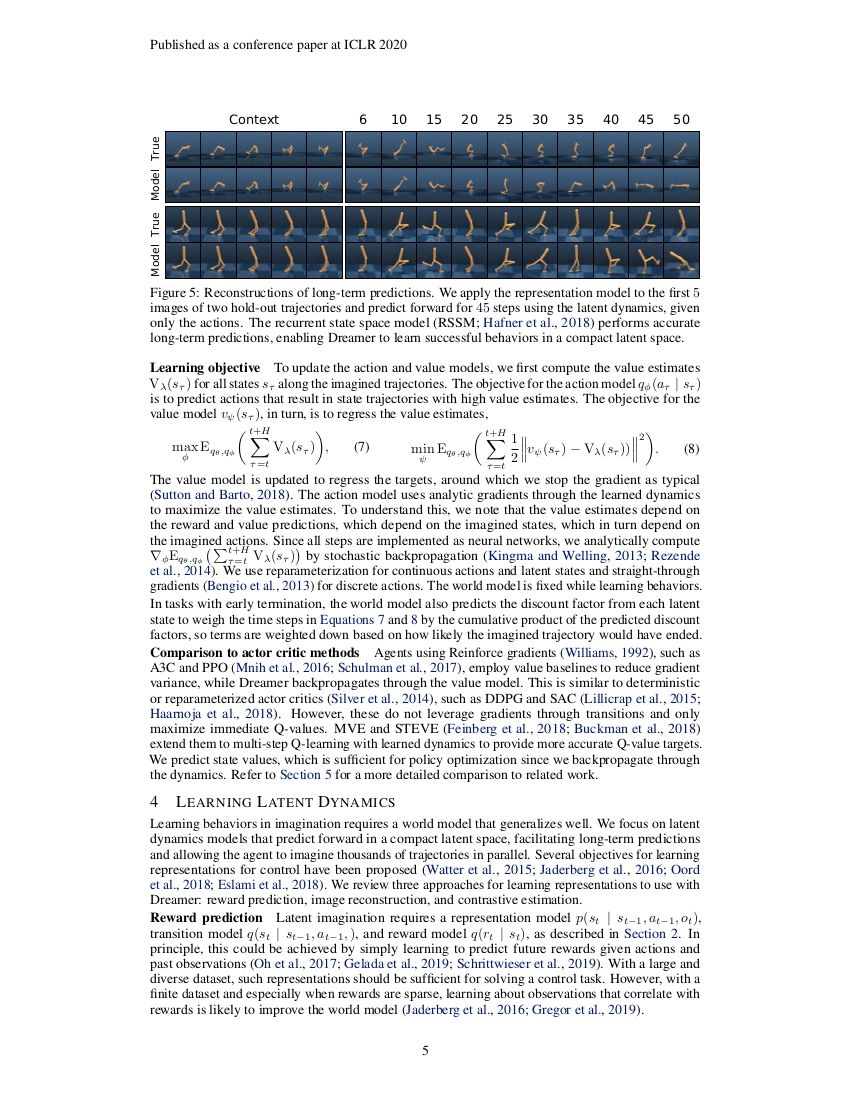
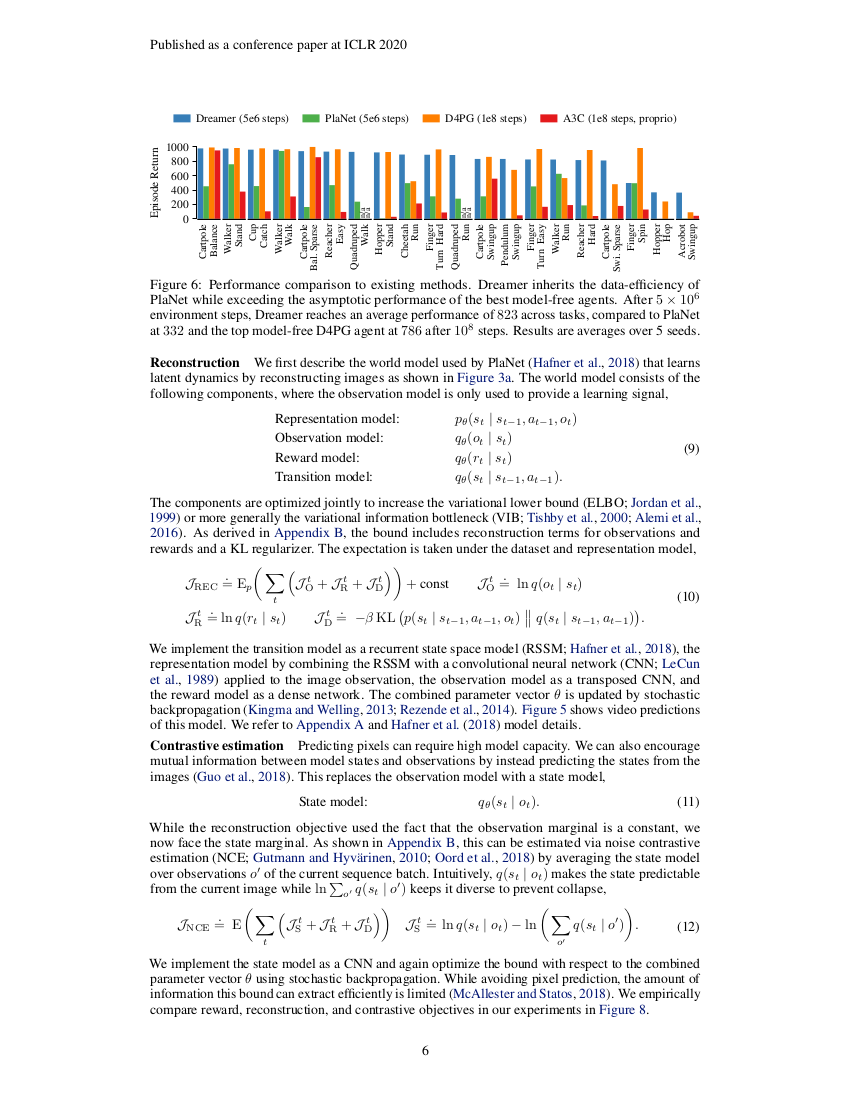
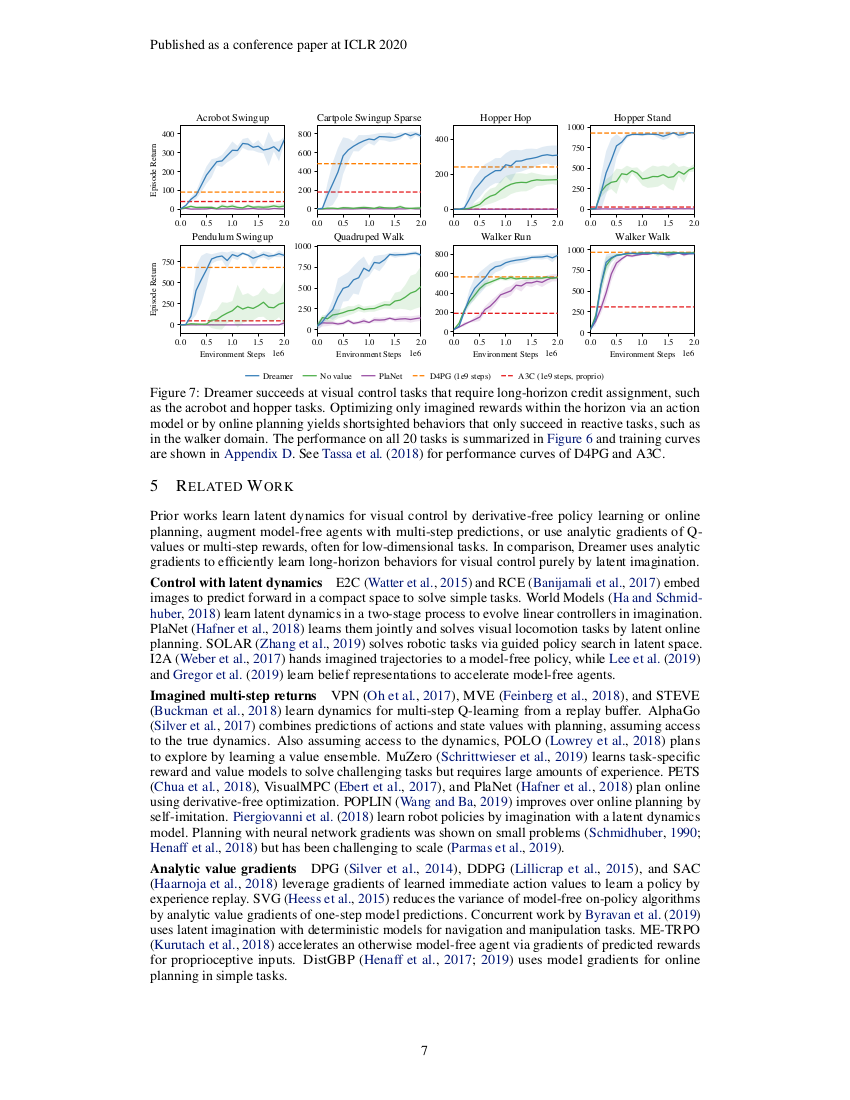
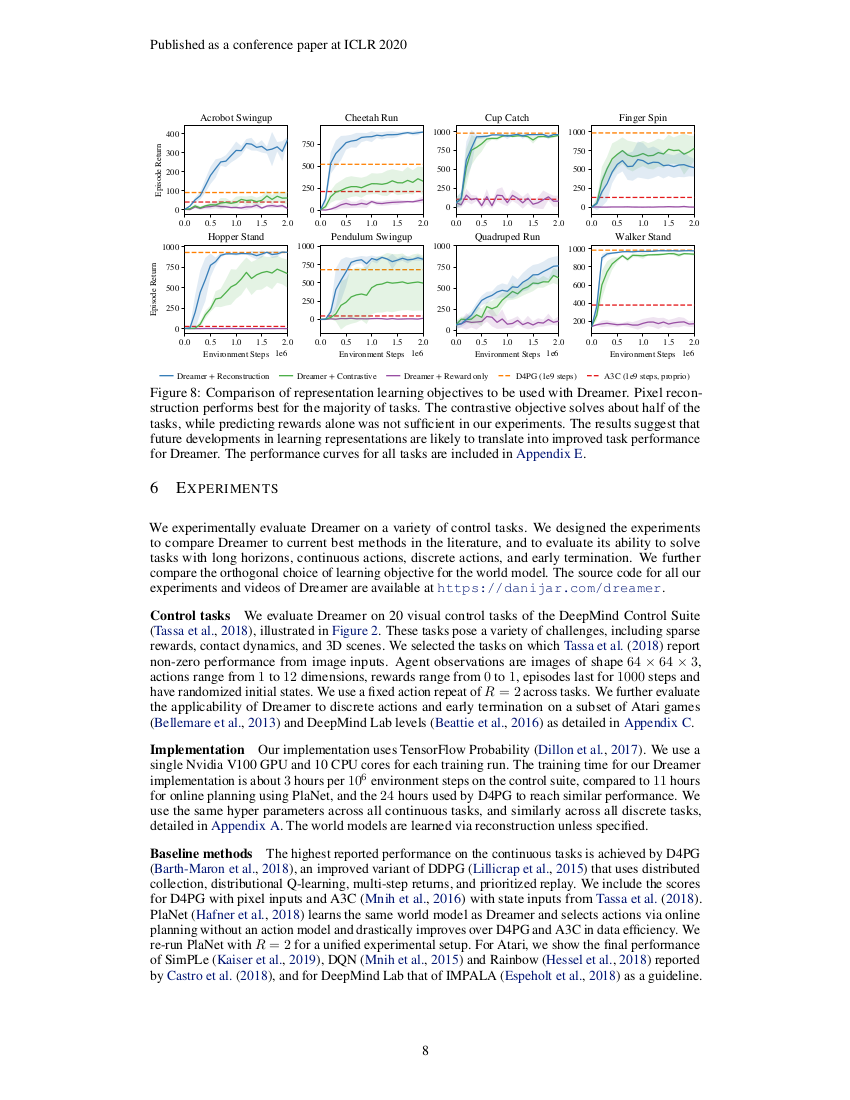
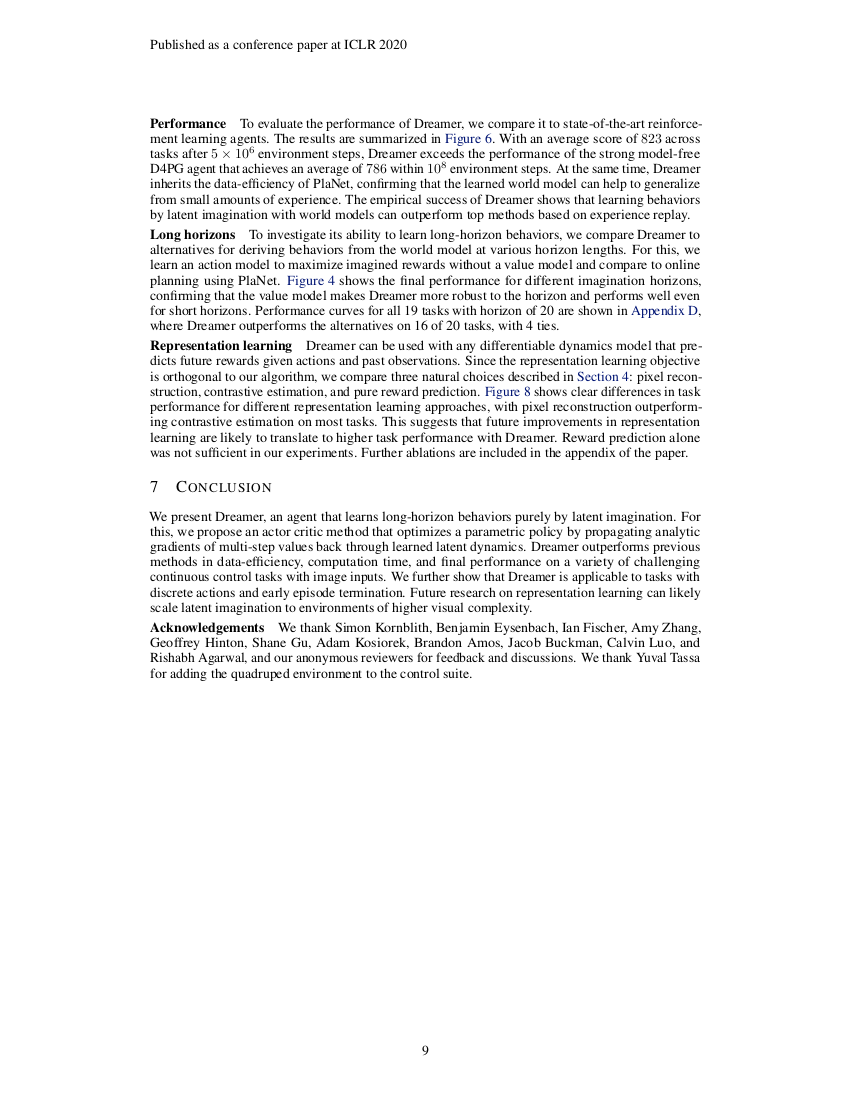